Lookalike modelling has been a staple of many marketing strategies for the last decade. But with stricter data privacy compliance requirements, changes in how browsers treat identity data, and a shift in consumer awareness of how their data is being used, traditional approaches to lookalike modelling are facing growing challenges.
What is lookalike modelling?
As your business grows, advertising plays an important role in attracting new customers to your brand. But advertising on mass can be very costly and result in wasted advertising spend. Lookalike modelling is a strategy that provides greater scale to advertising activity by identifying the common demographic and/or behavioural characteristics of your current customers, and targeting individuals that share these characteristics.
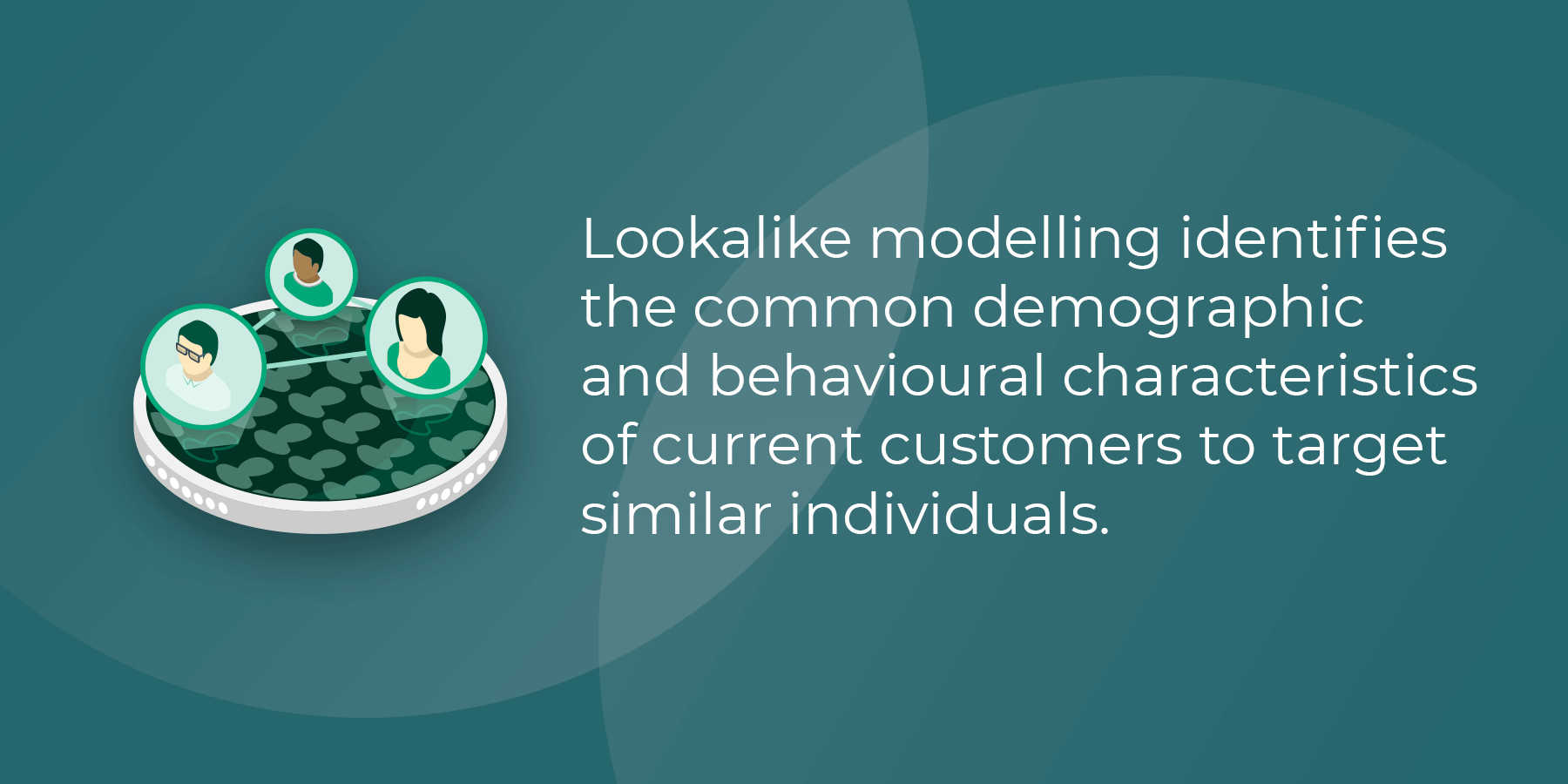
Let’s imagine you are a sports brand looking to launch your latest sneaker. A lookalike model will use your existing first-party data on customers who bought similar sneakers in the past, commonly known as a seed audience, and analyse the demographic and behavioural characteristics the individual’s exhibit. A very simple analysis might confirm that there is a high percentage of current customers who are female, aged 18-34 and enjoy running.
This profile is then used to identify audiences that share these particular characteristics, and therefore have a higher propensity to buy these new sneakers.
Traditional approaches to lookalike modelling
As with much of the current advertising ecosystem, traditional approaches to lookalike modelling rely heavily on the use of third-party cookies.
Currently, to achieve lookalike modelling, the sports brand mentioned above would place a third-party cookie on their website that would track the behaviours of website visitors, storing all this data in a central platform such as a DMP. Over time, this DMP would be able to identify the behaviours individuals share that are most likely to lead to a purchase.
Because DMPs are widely adopted across various brand and publisher sites and employ cross-site tracking techniques which track a user's behaviour from site to site, a DMP can find other individuals that share common characteristics with that seed audience, and target those lookalikes with an advertising campaign.
Challenges facing traditional approaches
The first challenge facing these traditional approaches is the accuracy they deliver. Because most approaches require data to be ‘flattened’ in order to conduct analysis across multiple datasets, the targeting tends to be based on pre-build segmentation. Continuing our above example, the sports brand would identify a high percentage of their customers that exist in a “running enthusiast” segment, and therefore they target that segment. This means many of the intricacies of what makes up a customer are lost and lookalike modelling becomes one-dimensional.
This segment-based approach also creates our second challenge, that lookalike modelling, for the most part, exists within a blackbox. If we take the above example, the sports company uses their DMP, who has run a lookalike model to identify the best segments to target. Those segments can then be targeted across a media owners audience. In that scenario, of the three parties involved, only the DMP knows how that segment has been created.
Finally, the majority of lookalike modelling solutions rely on third-party cookies. Because data collected by third-party cookies can be read across multiple domains, they enable the analysis of the seed audience behaviour across multiple sites, enabling them to identify the characteristics of those buyers. And because those third-party cookies exist across multiple publishers, the audience segments the lookalike model identifies as being “on target” can then be targeted across the internet.
With the depreciation of third-party IDs, lookalike modelling that is reliant on data gained from cross-site tracking of users is now, rightly, under threat of disappearing entirely.

InfoSum’s approach with a decentralised data clean room
Data clean rooms, and specifically decentralised data clean rooms such as that provided by InfoSum, provide a privacy-safe solution that delivers greater accuracy than traditional approaches.
Through a decentralised data clean room, multiple first, second and third-party data sources can be analysed, a lookalike model run and those individuals targeted, without any data being shared between those parties, and importantly, without any third-party IDs.
Continuing our previous example, the sports brand would upload their first-party customer data, full of rich behavioural and demographic knowledge, into a Bunker. A media owner would also upload their authenticated addressable audience into a Bunker. The sports brand can now run analysis on the intersection of their customers and the media owners audience. Through that analysis, they can identify the common attributes that make up that overlap.
Where needed, a third-party can also make their insight available for the sports brand and the media owner. This additional knowledge can be used to further interrogate the behavioural and demographic attributes that make up the intersection.
Unlike traditional approaches that rely on pre-built segments, through this next-generation approach, they can identify the specific attributes that their customers share. So instead of relying on a basic “running enthusiast” segment, they can learn there is a high percentage of gym runners, rather than road runners.

The sports brand and the media owner can then work together to identify the attributes, rather than the segments, they wish to target. The media owner can then look at the rest of their audience and use those attributes to create a custom audience for the sports brand to target.
Importantly, all of this happens with complete transparency for every party, but never exposes the underlying data between each party. Additionally, because we use differential privacy techniques, it is impossible for a single individual to ever be re-identified.
Data onboarding, the first step in lookalike modelling
If you’re looking to get started with lookalike modelling in the new privacy-first era, the first step is to select a data clean room solution that enables a privacy-by-design data onboarding solution. InfoSum offers the only decentralised end-to-end data onboarding solution that enables you to deliver relevant and timely marketing faster and at a greater scale than ever before.
Once your data has been onboarded with InfoSum, you are able to conduct seamless analysis across multiple media owners to identify where a natural synergy exists and lookalike modelling will be advantageous.